Project: Anima
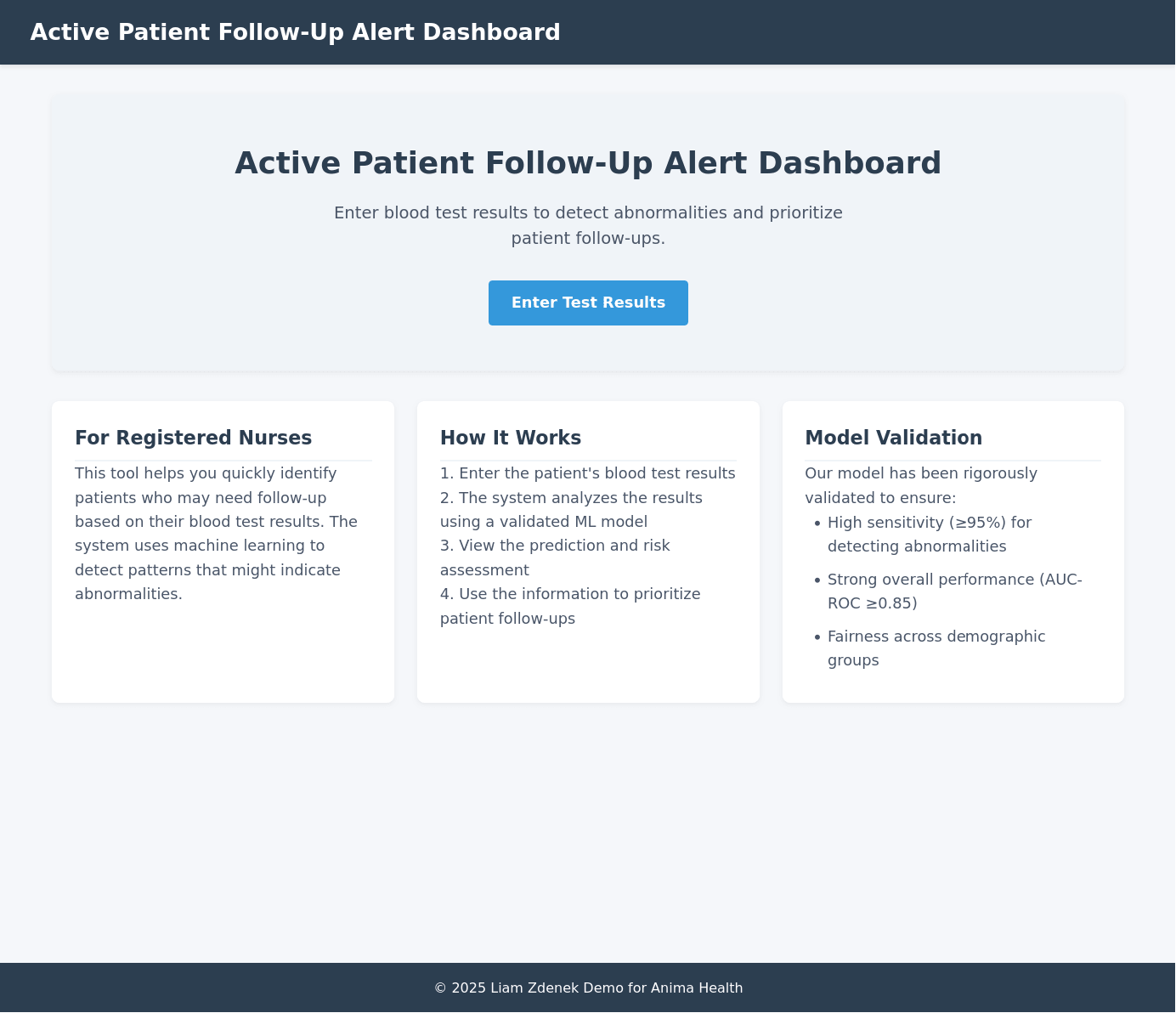
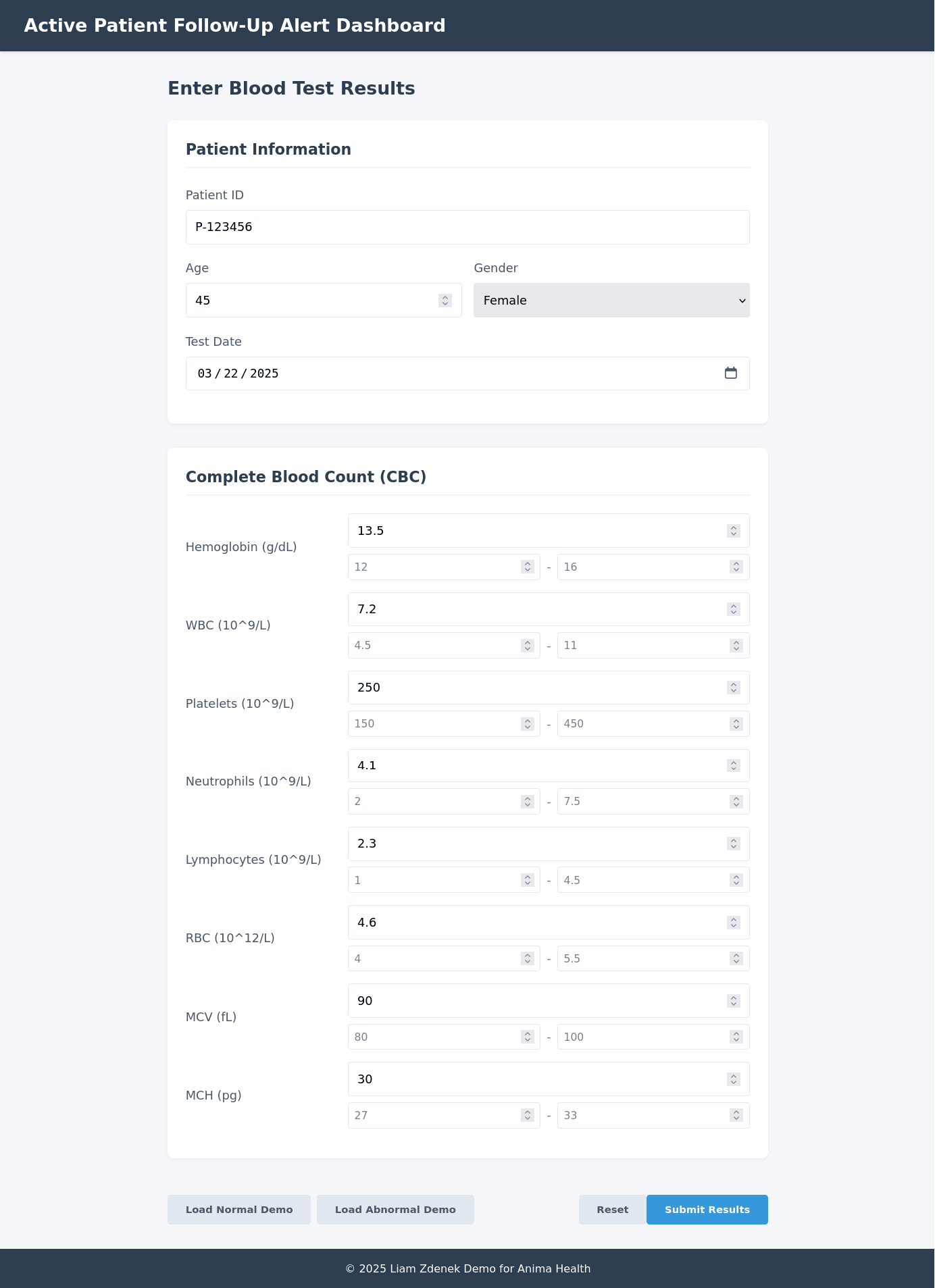
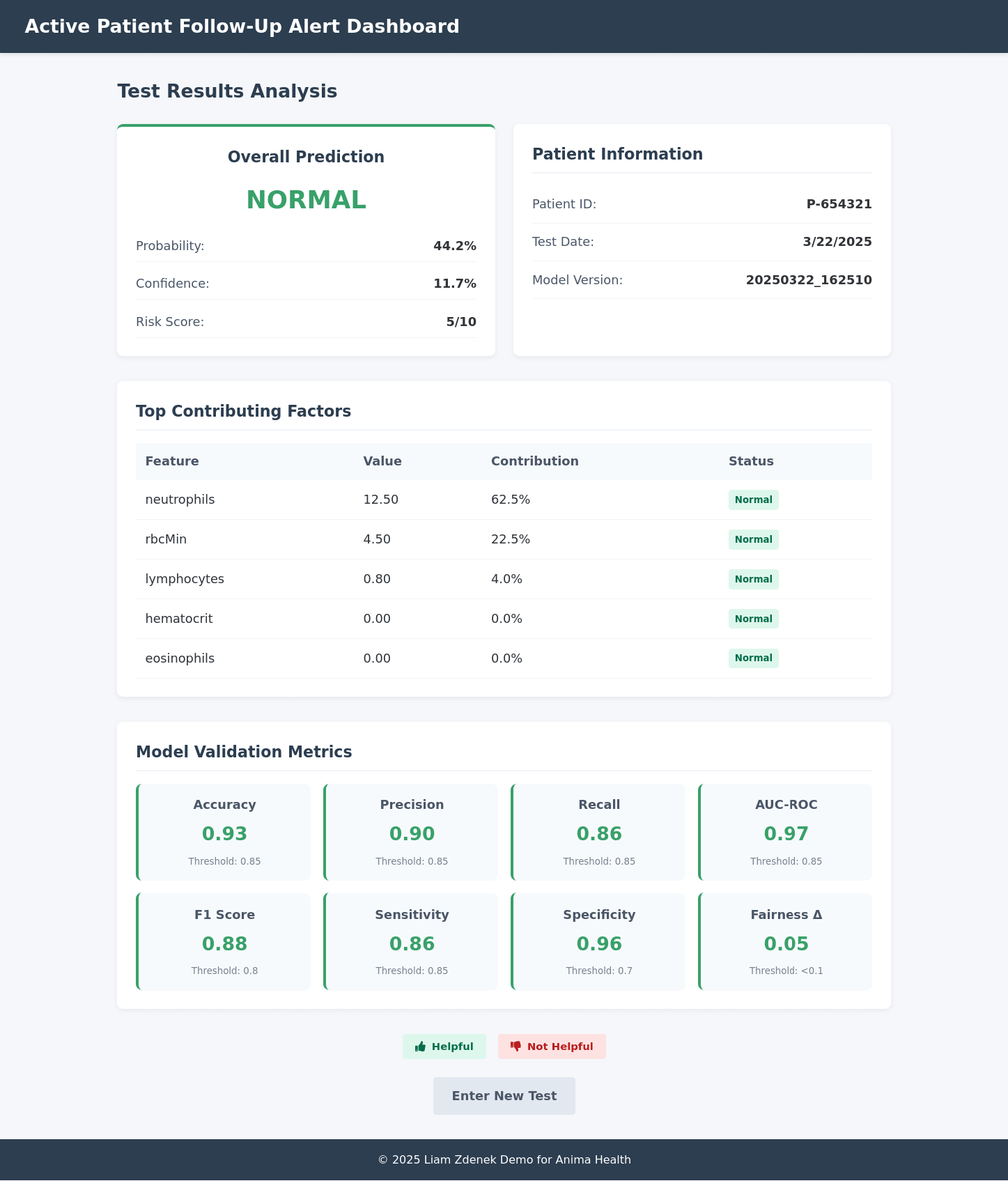
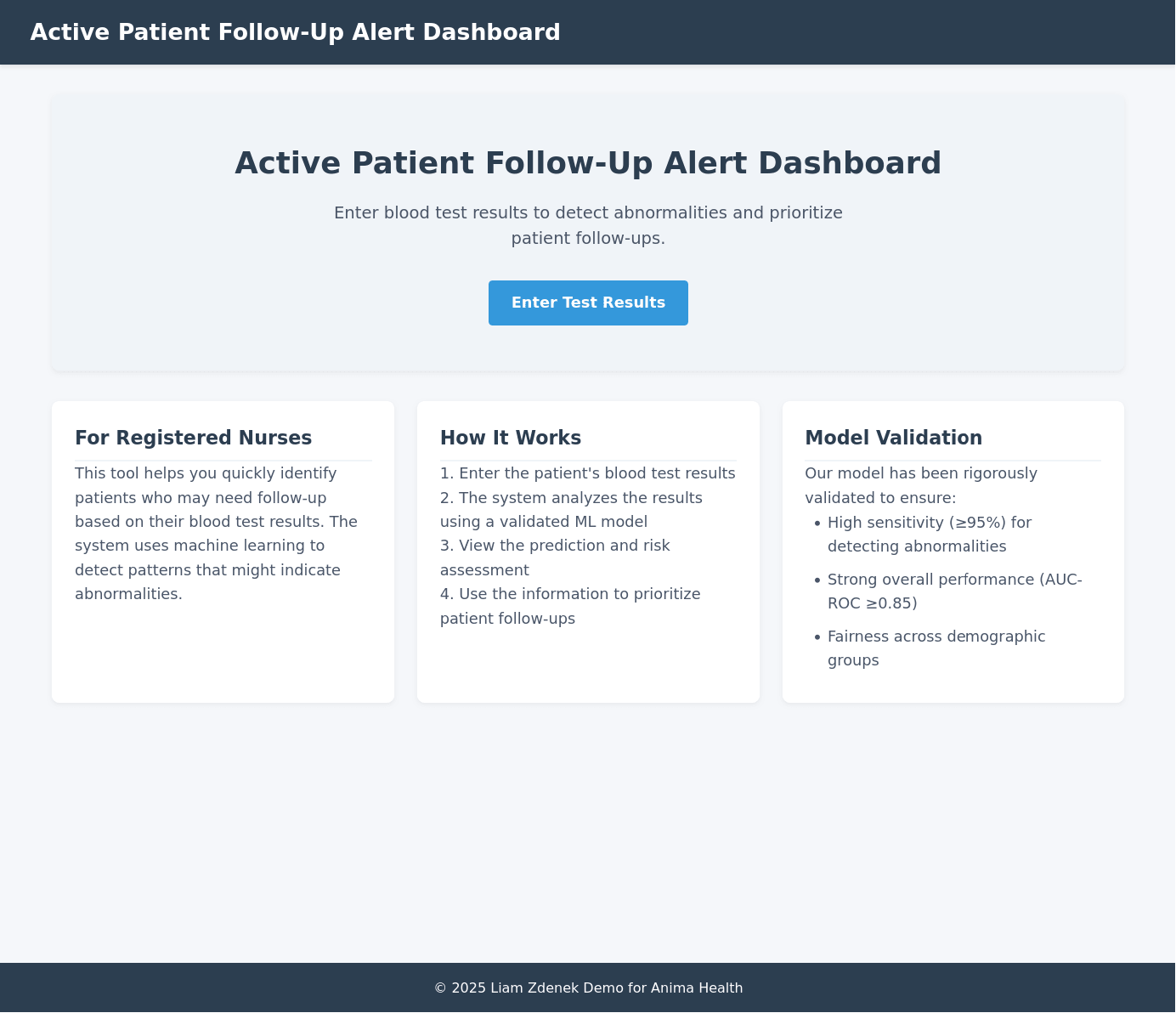
Active Patient Follow-Up Alert Dashboard
Anima
A lightweight web application that simulates an automated alert system for abnormal lab results with machine learning-based risk scoring.
Project Demo
Project Metrics
Project Details
The Active Patient Follow-Up Alert Dashboard uses machine learning to help clinicians identify and prioritize abnormal lab results that require follow-up. It implements an active learning feedback loop to continuously improve detection accuracy and reduce alert fatigue.
Business Value
- Patient Safety
- Clinical Decision Support
- Workflow Optimization
Key Features
Abnormal Lab Result Detection
Identifies patients with abnormal blood test results that require clinical follow-up and prioritizes patients based on risk scores.
Machine Learning Pipeline
Trains models on synthetic blood test data, validates models for clinical safety and fairness, and serves predictions via a REST API.
Active Learning Feedback Loop
Incorporates clinician feedback to continuously improve the alert algorithm and adapt to real-world usage patterns.
User Interface
Allows healthcare providers to enter blood test results and displays prediction results with risk scores and contributing factors.
Technologies Used
Frontend
Backend
DevOps
Other
Challenges & Solutions
Synthetic data may not capture all real-world patterns
Enhanced data simulator with more complex abnormality patterns and demographic-specific reference ranges.
Potential overfitting on synthetic data
Implemented robust cross-validation, regularization, and temporal validation.
Limited feature engineering
Created derived features based on reference ranges and clinical relevance.
Key Learnings
- Clinical Safety Priority: In healthcare applications, minimizing false negatives (missed abnormal results) is often more important than reducing false positives.
- Validation Complexity: Healthcare ML models require comprehensive validation beyond standard metrics, including fairness across demographics and temporal stability.
- Active Learning Value: Incorporating user feedback creates a continuously improving system that adapts to real-world usage patterns.
- Synthetic Data Limitations: While synthetic data is valuable for development and testing, it has limitations in capturing the full complexity of real-world clinical data.